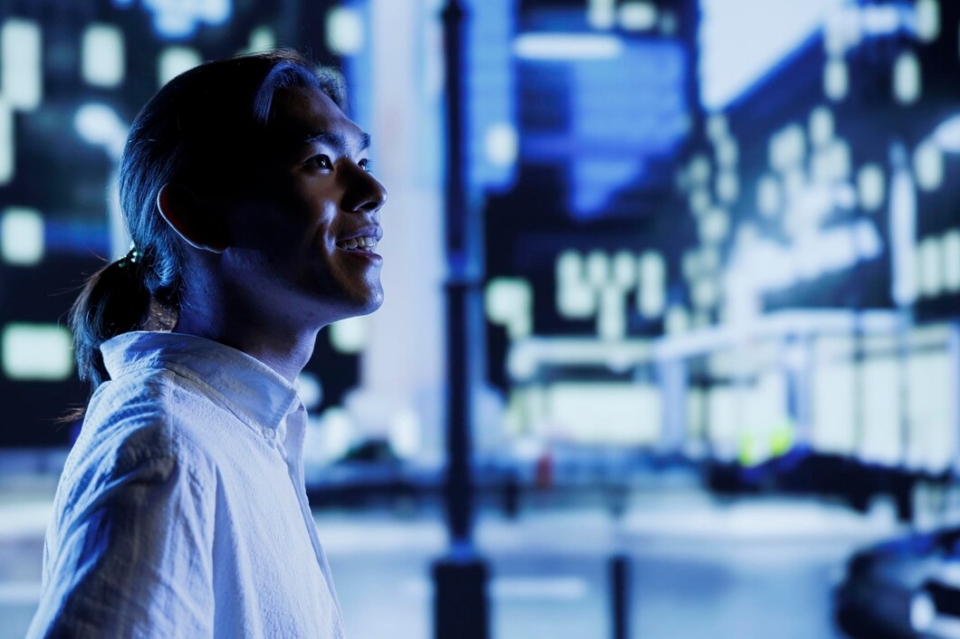
Today’s businesses regularly seek ways to gain competitive advantages through innovation. In a recent Pipefy survey of business leaders, 81% of C-suite executives report that they expectgenerative AI adoption to improve efficiency at their businesses by more than 25%. Enterprise AI has the potential to bring this to fruition, transforming large organizations’ operations, decision making, and customer service strategies.
Enterprise AI employes sophisticated machine learning (ML) models that have fundamentally changed the discipline of data science to one that maximizes the efficiency of business processes. This article examines the benefits and applications of enterprise AI for business leaders seeking new means to make better, data-driven decisions faster.
Access Pipefy’s free Automation Trends Report now
What is enterprise AI?
Enterprise AI is software that employs advanced artificial intelligence (AI) techniques like ML to drive digital transformation. Large organizations use it to achieve significant improvements in their business processes, resulting in higher operational efficiency, greater profitability and increased business agility.
Driving factors in the development of enterprise AI include recent specific AI innovations, cloud computing, big data and the internet of things (IoT).
AI vs. enterprise AI
Enterprise AI differs from the AI technology formats we commonly encounter in its scale; it must function effectively within the existing complex operating environments of large organizations. Its size and complexity are not the only differentiating features, however, as it must also align with an organization’s broad objectives in several different ways, namely:
Scalability
Enterprise AI systems must be powerful enough to continually process data sets of all sizes and expand their business operations in response to growing needs, without significant redesign. This scalability typically refers to employee/user number, data quality and data complexity.
Sustainability
In the landscape of enterprise AI, sustainability refers to the ability to maintain efficient operations over the long term. For example, an enterprise AI system that ensures consistent on-time deliveries yields satisfied customers, minimal rework costs, and the inevitable impact of delays on subsequent projects – sustainability on multiple levels.
In addition, sustainability indicates AI platforms and operations that emphasize the conservation of resources for a better impact on the environment, including the ability to adapt to technological advancements that further those values.
Governance
Governance in enterprise AI is the practice of establishing policies for managing processes. The most effective operational processes are those that are fully transparent to all with a clear path of accountability.
Another critical aspect of AI enterprise governance is an organization’s responsibility to set high legal and ethical standards, placing particular emphasis on maintaining the accountability of AI-based decision-making.
Integration capacity
Every business employs systems it considers non-negotiable for daily work -such as ERPs or time-tracking software. AI technology must seamlessly interact with and, in some cases, broaden the use of these existing systems and applications. When it does, the smooth flow of data and interoperability throughout the enterprise’s IT infrastructure improves the overall effectiveness and efficiency of operations.
Reliability
Reliability is an attribute that few appreciate, let alone notice, until it vanishes. Enterprise AI must perform consistently with minimal downtime under a large variety of conditions, including the risks posed by human errors, system failures, and long repair times. Continued accuracy and system availability are non-negotiable aspects of any enterprise AI software.
Value
Enterprise AI should provide tangible benefits for the organization. These commonly include cost savings, increased operational efficiency, improved user experiences and the identification of new revenue opportunities.
Security
Much of the data maintained by enterprises is sensitive, so an essential element of enterprise AI systems is robust security. This requirement includes maintaining the confidentiality and integrity of data, secure user access and a strong defense against threats.
Flexibility
An AI system must adapt to changes in an organization’s objectives and fluctuating requirements. Common examples of flexibility include the ability to continue supporting multiple business functions in response to changes in organization or market trends.
Ease of use
User-friendliness is a crucial feature of enterprise AI solutions, as they must remain accessible and understandable to many types of users. In particular, enterprise AI should be useful to users who lack a strong technical background.
Enterprise AI benefits
The benefits of AI for an enterprise include:
- Scalability
- Personalization
- Cost savings
- Risk management
Scalability
Enterprise AI systems should easily scale in response to rapid changes in data and transaction volume. This trend most often occurs when an organization’s processes evolve or its operations grow, but as global and market events of the past five years show, scaling down and adapting to new work patterns is also crucial.
Personalization
AI algorithms customize the user experience by identifying patterns and predicting customer behavior, allowing them to make data-driven decisions about the experience they provide.
This process typically involves the analysis of large amounts of customer data in real-time, including browsing history, demographics, purchase patterns and social media interactions. AI systems can thus gain valuable insights into customer preferences, allowing businesses to deliver tailored experiences throughout each user’s journey.
Cost savings
Businesses of all sizes must strive to reduce operational costs, regardless of their current success. AI systems can automate routine tasks, reducing the frequency of human errors. In addition, enterprise AI can cut operating costs with predictive maintenance, thus saving on maintenance expenses and minimizing downtime.
Reducing operational costs is important for businesses of all sizes, regardless of their success. Automating routine tasks and processes reduces human errors and operational costs. However, this is not the only way enterprise AI can reduce your organization’s costs. For example, predictive maintenance minimizes downtime and saves on long-term maintenance costs.
Risk management
Enterprise AI can mitigate risk in a number of key areas, such as predicting the probability of a borrower defaulting on a loan. In addition, banks have long used data such as payment history to detect suspicious transactions that could indicate fraud. They also monitor traders by linking information on trades with behaviors such as e-mails and phone calls.
In supply chain management, AI solutions reduce risks by integrating supplier information such as geographical location and political environment when determining which supplier to use for a particular requirement.
Furthermore, the systems can manage risks by identifying software with characteristics that indicate malicious intent, even when the specific software is unfamiliar.
Is enterprise AI safe?
The ability of enterprise AI to customize interactions and services to individual needs can be highly beneficial, but implementing such a system requires careful consideration about its risks. For example, proper design and monitoring is essential for minimizing an AI system’s potential for bias or unethical use.
Data security and privacy is also a major factor due to the large amounts of sensitive data these systems are capable of handling for enterprises. Another area of concern is the effect that enterprise AI can have on the workforce. The increase in workflow efficiency can make some roles redundant, often requiring an enterprise to re-skill workers it wishes to retain.
Enterprise AI use cases
Enterprise AI drives decision-making and operational efficiency in many industries, including IT, procurement and HR.
IT
The ability of AI to analyze large amounts of data for the purpose of identifying trends and anomalies gives it many applications in IT. For example, enterprises can use AI to forecast changes in the demand for computing resources, hardware inventory and threat detection. Predictive analytics can now do the work of an entire team of data scientists using older methods to obtain data insights.
Procurement
Supply chain management has become increasingly important in today’s global economy, especially since the COVID-19 pandemic and its ripple effects on the market. Enterprise AI can optimize procurement operations by predicting fluctuations in demand, optimizing logistics routes and improving inventory management.
In addition, predictive maintenance helps prevent equipment breakdowns, maintain inventory at the proper level and minimize downtime.
HR
Enterprise AI is effective in many HR tasks, such as the use of AI to drive recruitment efforts. For example, these tools can conduct initial screenings and match candidates to suitable positions.
Chatbots, one of the most popular AI formats businesses currently use, can answer a large number of employee questions and issues with no human interaction, leaving HR professionals to tackle larger and more nuanced issues before they become problems.
Furthermore, enterprise AI can analyze employee sentiments, allowing an organization to gauge their level of satisfaction and identify areas for morale improvement. This capability helps retain employees by keeping them engaged with their work, which is more cost-effective than recruiting.
Find out how AI can revolutionize HR services in Pipefy’s guide The Next Era of HR
How to implement enterprise AI at your business
The implementation of enterprise AI consists of six steps that, when thoroughly observed and executed, have the potential to yield the operational cost savings, decision-making flexibility, and customization your business needs to outperform competitors.
- Define clear goals
The first step is to define the goals your business expects and/or hopes to achieve with AI software, such as improved operational efficiency, better customer experience or increased revenue. The better understanding leaders have of these objectives, the more effective the AI strategy will be, ensuring the implementation aligns with the organization’s overall objectives.
- Assign a multidisciplinary team
A cross-functional team is essential for providing the many skills needed to implement enterprise AI, including AI experts, data scientists and domain specialists. Such a team also supports a holistic approach to implementation, in which members combine their different areas of expertise and perspectives.
- Ensure the business has the necessary infrastructure and governance
An organization should already have the IT infrastructure resources needed to support an enterprise AI implementation. It should also have the proper data governance process in place before implementing such a solution.
- Devise and launch a pilot program
Some Small organizations can successfully implement AI solutions for their entire infrastructure at once, but enterprises should launch a pilot program first. This approach allows the organization to test various solutions in a controlled environment, identifying potential issues and bottlenecks before scaling up.
- Integrate with processes and scale
Once the pilot program is successful, an organization should integrate the selected AI technology into all existing workflows. This step requires careful planning to ensure systems are compatible and to minimize disruption to current operations.
- Continuously monitor and improve
When the full implementation is complete, enterprises should continuously monitor their AI systems. This includes regular updates designed to improve AI performance, especially in response to evolving business needs.
Overcoming common enterprise AI implementation challenges
Enterprise AI tools are designed to make businesses more efficient, but implementing them has its own set of challenges. Whether they simply don’t understand the technology or are unable to gain the trust of users, business leaders can face a number of struggles when integrating AI into their processes. These barriers to adoption include maintenance, data security concerns, ethical and legal responsibilities and change management.
Maintenance
The success of AI solutions relies on the quality of the data the software harvests and uses. It often comes from multiple sources, making its accuracy and relevance a primary consideration when maintaining enterprise AI. This ongoing process also requires a workforce with expertise in multiple disciplines, including AI, data science and ML. Maintenance teams often require upskilling, unless an organization hires external experts in these fields.
AI can realize long-term cost savings for an organization, but the company must make initial investments in the technology. In addition to the cost of the solution itself, AI may also require a substantial investment in infrastructure and training. An enterprise must therefore weigh these costs against the expected benefits of AI.
Data security concerns
Data security concerns are one of the biggest challenges businesses face when implementing AI. These models generally require large data sets to learn and adapt their behavior, but handling this sensitive information also poses serious risks for enterprises.
Organizations must comply with the various data protection regulations that require and instruct them to prevent potential breaches. As a result, enterprises need to stay up-to-date on data protection trends to ensure they can handle emerging threats.
Ethical and legal responsibilities
AI is evolving rapidly, making it difficult to regulate. This issue is further complicated by the addition of related technologies like ML and deep learning, so regulatory bodies throughout the world are actively working to develop rules for using enterprise AI.
In addition to the current lack of recognized standards, the many divergent legal frameworks create considerable ambiguity regarding compliance with legal requirements.
However, some legislation already exists, such as the General Data Protection Regulation (GDPR). This legislation mandates strict data protection measures in the European Union (EU), including anonymization and encryption techniques that still allow for meaningful AI analysis.
Change management
Introducing AI to an organization involves changes for people as well as technology. Employees are likely to be concerned about their jobs and the need to learn new skills, in addition to the frustration of changing workflows. Businesses must directly address this issue before employees become resistant to an AI implementation, which can quickly sabotage an AI initiative.
What is the future of enterprise AI?
The development of generative AI technology has fundamentally changed enterprise AI across many business domains. Its ability to use large language models (LLMs) to create new solutions will soon extend beyond the current predictive models.
In addition, generative AI can already create highly personalized content for marketing copy, programming code and other creative solutions to complex problems. The most significant developments in AI for the near future will likely include increased automation for more complex tasks, such as predicting market trends and customer behavior.
Start employing enterprise AI with Pipefy
Implementing enterprise AI requires the right tools and a deep understanding of the methodologies it employs, including cloud-computing frameworks, data science and deep learning. Knowledge of the specific business domain is also a key requirement for a successful AI solution, requiring a team of subject-matter experts, programmers and data scientists who can develop and maintain these systems.
Pipefy is a trusted partner with experience integrating AI into your existing infrastructure, allowing your organization to get the maximum value from its AI investment.
Our dedicated platform creates business process models (BPMs) and optimizes them for your business. These solutions also offer features like user alerts and tagging to facilitate the hand-over between process steps. They also help you make smarter decisions about business processes by analyzing historical data.